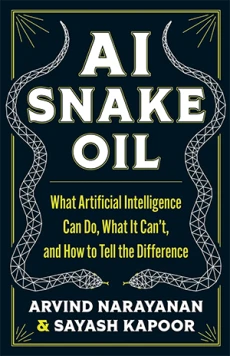
AI Snake Oil: What Artificial Intelligence Can Do, What It Can’t, and How to Tell the Difference
Arvind Narayanan & Sayash Kapoor
360 pages, Princeton University Press, 2024
Imagine an alternate universe in which people don’t have words for different forms of transportation, only the collective noun “vehicle.” They use that word to refer to cars, buses, bikes, spacecraft, and all other ways of getting from place A to place B. Conversations in this world are confusing. There are furious debates about whether or not vehicles are “environmentally friendly,” but (even though no one realizes it) one side of the debate is talking about bikes and the other side about trucks. There is a breakthrough in rocketry, but when the media focuses on how vehicles have gotten faster, people call their car dealer (oops, vehicle dealer) to ask when faster models will be available. Meanwhile, fraudsters have capitalized on the fact that consumers don’t know what to believe when it comes to vehicle technology, so scams are rampant in the vehicles sector.
Now replace the word “vehicles” with “artificial intelligence,” and we have a pretty good description of the world we live in.
Artificial Intelligence, AI for short, is an umbrella term for a set of loosely related technologies. ChatGPT has little in common with, say, software that banks use to evaluate loan applicants. Both of these are referred to as AI, but in all the ways that matter—how they work, what they’re used for and by whom, and how they fail—they couldn’t be more different.
Chatbots, as well as image generators like Dall-E, Stable Diffusion, and Midjourney, fall under the banner of what’s called generative AI. Generative AI can generate something in seconds: Chatbots generate often realistic answers to human prompts and image generators produce photorealistic images matching almost any description, say “a cow in a kitchen wearing a pink sweater.” Other apps can generate speech or even music.
Generative AI has been rapidly advancing, its progress genuine and remarkable. But as a product, it is still immature, unreliable, and prone to misuse. At the same time, its popularization has been accompanied by hype, fear, and misinformation.
Still, we’re cautiously optimistic about the potential of this type of AI to make people’s lives better in the long run. Predictive AI is a different story.—Arvind Narayanan & Sayash Kapoor
* * *
In the last few years, applications of predictive AI to predict social outcomes have proliferated. Developers of these applications claim to be able to predict future outcomes about people, such as whether a defendant would go on to commit a future crime or whether a job applicant would do well at a job. In contrast to generative AI, predictive AI often does not work at all. People in the United States over the age of sixty-five are eligible to enroll in Medicare, a state-subsidized health insurance plan. To cut costs, Medicare providers have started using AI to predict how much time a patient will need to spend in a hospital. These estimates are often incorrect. In one case, an eighty-five-year-old was evaluated as being ready to leave in seventeen days. But when the seventeen days passed, she was still in severe pain and couldn’t even push a walker without help. Still, based on the AI assessment, her insurance payments stopped. In cases like this, AI technology is often deployed with sensible intentions. For example, without predictive AI, nursing homes would be logically incentivized to house patients forever. But in many cases, the goals of the system as well as how it’s deployed change over time. One can easily imagine how Medicare providers’ use of AI may have started as a way to create a modicum of accountability for nursing homes, but then morphed into a way to squeeze pennies out of the system regardless of the human cost.
Similar stories are prevalent across domains. In hiring, many AI companies claim to be able to judge how warm, open, or kind someone is based on their body language, speech patterns, and other superficial features in a thirty-second video clip. Does this really work? And do these judgments actually predict job performance? Unfortunately, the companies making these claims have failed to release any verifiable evidence that their products are effective. And we have lots of evidence to the contrary, showing that it is extremely hard to predict individuals’ life outcomes, as we’ll see in chapter 3.
In 2013, Allstate, an insurance company, wanted to use predictive AI to determine insurance rates in the U.S. state of Maryland—so that the company could make more money without losing too many customers. It resulted in a “suckers list”—a list of people whose insurance rates increased dramatically compared to their earlier rates. Seniors over the age of sixty-two were drastically overrepresented in this list, an example of automated discrimination. It is possible that seniors are less likely to shop around for better prices and that AI picked up on that pattern in the data. The new pricing would likely increase revenue for the insurance company, yet it is morally reprehensible. While Maryland refused Allstate’s proposal to use this AI tool on the grounds that it was discriminatory, the company does use it in at least ten other U.S. states.
If individuals object to AI in hiring, they can simply choose not to apply for jobs that engage AI to judge résumés. When predictive AI is used by governments, however, individuals have no choice but to comply. (That said, similar concerns also arise if many companies were to use the same AI to decide who to hire.) Many jurisdictions across the world use criminal risk prediction tools to decide whether defendants arrested for a crime should be released before their trial. Various biases of these systems have been documented: racial bias, gender bias, and ageism. But there’s an even deeper problem: evidence suggests that these tools are only slightly more accurate than randomly guessing whether or not a defendant is “risky.”
One reason for the low accuracy of these tools could be that data about certain important factors is not available. Consider three defendants who are identical in terms of the features that might be used by predictive AI to judge them: age, the number of past offenses, and the number of family members with criminal histories. These three defendants would be assigned the same risk score. However, in this example, one defendant is deeply remorseful, another has been wrongly arrested by the police, and the third is itching to finish the job. There is no good way for an AI tool to take these differences into account.
Another downside of predictive AI is that decision subjects have strong incentives to game the system. For example, AI was used to estimate how long the recipient of a kidney transplant would live after their transplant. The logic was that people who had the longest to live after a transplant should be prioritized to receive kidneys. But the use of this prediction system would disincentivize patients with kidney issues to take care of their kidney function. That’s because if their kidneys failed at a younger age, they would be more likely to get a transplant! Fortunately, the development of this system involved a deliberative process with participation by patients, doctors, and other stakeholders. So, the incentive misalignment was recognized and the use of predictive AI for kidney transplant matching was abandoned.
Are things likely to improve over time? Unfortunately, we don’t think so. Many of its flaws are inherent. For example, predictive AI is attractive because automation makes decision-making more efficient, but efficiency is exactly what results in a lack of accountability. We should be wary of predictive AI companies’ claims unless they are accompanied by strong evidence.